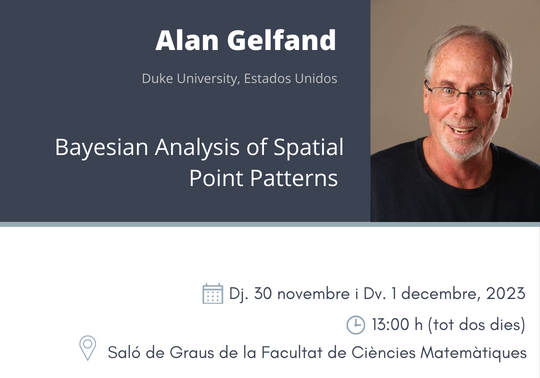
Alan Gelfand: "Bayesian Analysis of Spatial Point Patterns"
Data i lloc: Dijous 30 de novembre i divendres 1 de decembre de 2023 · 13:00 h · Saló de Graus de la Facultat de Ciències Matemàtiques
Resum: Spatial point patterns arise in many contexts. Examples include: ecological processes, e.g., the pattern of trees in a forest; spatial epidemiology, pattern in disease cases, perhaps different patterns for cases vs. controls; syndromic surveillance to identify disease outbreaks, e.g., clustering of cases; the evolution/growth of a city, i.e., urban development.
There is a rich probabilistic literature on stochastic process modeling for point patterns. With regard to spatial point patterns, more recently, there has been consequential attention paid to the applied side - fitting of models and data analysis.
From the Bayesian perspective, this area of spatial analysis has lagged in development behind geostatistical/point referenced effort using Gaussian processes and the lattice/areal unit effort using Markov random fields. However, recently, we have seen substantial progress in Bayesian fitting of spatial point pattern models. Given such models can be fitted, the contribution here concerns development of a strategy for Bayesian inference with regard to model adequacy, model comparison, and full inference under a selected model.
Our approach rests on posterior simulation, as in much of current Bayesian analysis. Here, we need posterior simulation of point patterns from which we can extract posterior inference for features. For us, the bottom line is: if you can simulate point patterns, you can carry out the foregoing inference. Our work draws upon variants of the Georgii-Nguyen-Zessin (GNZ) formula which, for us, immediately supplies posterior Monte Carlo integrations for many features of interest. We also offer an iterated expectation version. We illustrate the above using examples involving nonhomogeneous Poisson processes, log Gaussian Cox processes, and Strauss processes.