Deep learning will need Physics to solve the complexity of Earth sciences
- Fundació Parc Científic
- February 19th, 2019
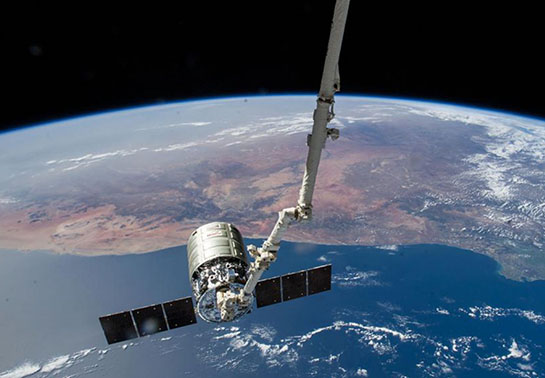
A collaborative study between the Max Planck Institute, the Universitat de València and the Lawrence Berkeley National Laboratory (USA) was just published in the journal Nature. The work shows that machine learning and deep learning in particular can substantially improve our understanding of the climate and the Earth system. The potential of deep learning has only partially been exploited so far in Earth sciences. In particular, complex dynamic processes such as hurricanes, fire propagation, and vegetation dynamics can be better described with the help of modern machine learning. As a result, climate and Earth system models will be improved, with new models combining artificial intelligence and physical modeling.
In the past decades mainly static attributes have been investigated using machine learning approaches, such as the distribution of land and vegetation properties from local to global scales. For some time now, it has been possible to tackle more dynamic processes by using more sophisticated deep learning techniques. This allows for example to quantify the global photosynthesis on land with simultaneous consideration of seasonal and short term variations.
Deducing underlying laws from observation data
“From a plethora of sensors, a deluge of Earth system data has become available, but so far we've been lagging behind in analysis and interpretation,” explains Markus Reichstein, managing director of the Max Planck Institute for Biogeochemistry in Jena, and first author of the publication. Examples for application are extreme events such as fire spreads or hurricanes, which are very complex processes influenced by local conditions but also by their temporal and spatial context. This also applies to atmospheric and ocean transport, soil movement, and vegetation dynamics, some of the classic topics of Earth system science.
Artificial intelligence to improve climate and Earth system models
However, direct application of deep learning approaches to Earth system problems is challenging. All data-driven and statistical approaches do not guarantee physical consistency per se, are highly dependent on data quality, and may experience difficulties with extrapolations. Besides, the requirement for data processing and storage capacity is very high. The publication discusses all these requirements, challenges and obstacles, and develops a strategy to efficiently combine machine learning with physical modeling. If both techniques are brought together, so-called hybrid models are created. They can for example be used for modeling the motion of ocean water to predict sea surface temperature. While the temperatures are modelled physically, the ocean water movement is represented by a machine learning approach. “The idea is to combine the best of two worlds, the consistency of physical models with the versatility of machine learning, to obtain greatly improved models,” Markus Reichstein further explains.
Prof. Gustau Camps-Valls, coordinator of the Image and Signal Processing (ISP) group from the Universitat de València adds “Machine learning plays a significant role nowadays in fields where the data shows strong temporal or spatial correlations, like in image, video or speech processing: algorithms learn that structure very well and can do inferences (predictions) with outstanding accuracy.” The problem comes in two ways: first, very often models are so complex that they become a black box and interpretability is compromised; and second, when these models are analyzed in depth they do not respect fundamental laws of Physics like energy or mass conservation. “The latter is really annoying. This is why we posit in this paper that data-driven and physics-aware machine learning is the way forward. This way Physics laws are fulfilled and observations are taken into account.”, says Prof. Camps-Valls. In a way, such hybrid models are intended to reconcile two communities.
The scientists contend that detection and early warning of extreme events as well as seasonal and long-term prediction and projection of weather and climate will strongly benefit from the discussed deep-learning and hybrid modelling approaches.
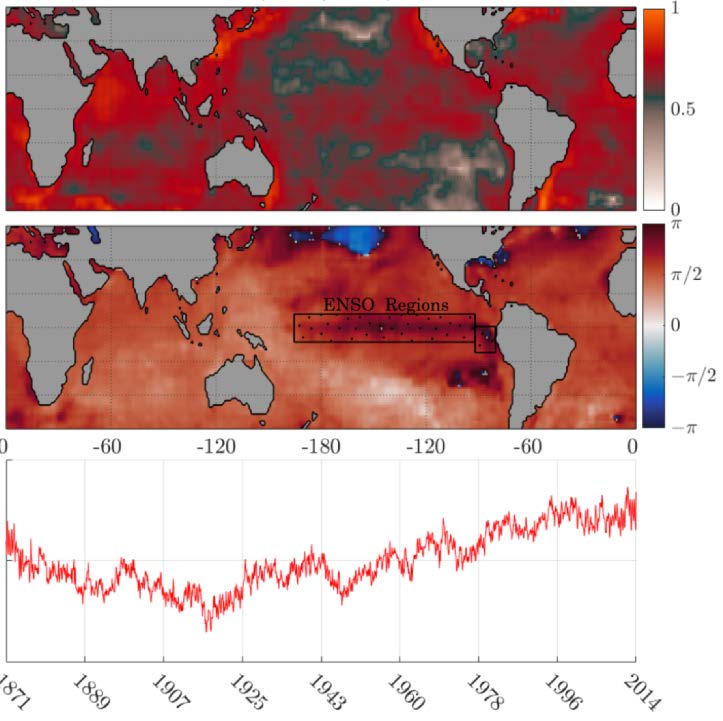
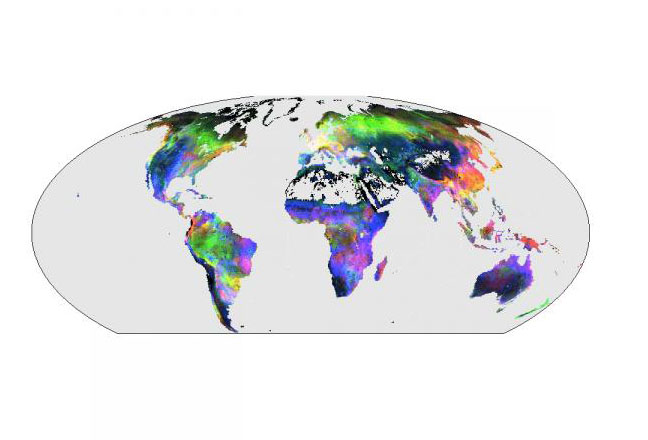
Original publication
«Deep learning and process understanding for data-driven Earth system science»
Reichstein M., Camps-Valls G., Stevens B., Jung M., Denzler J. Cavalhais N., Prabhat (2019)
Nature 566, 195-204, doi: 10.1038/s41586-019-0912-1
More information: