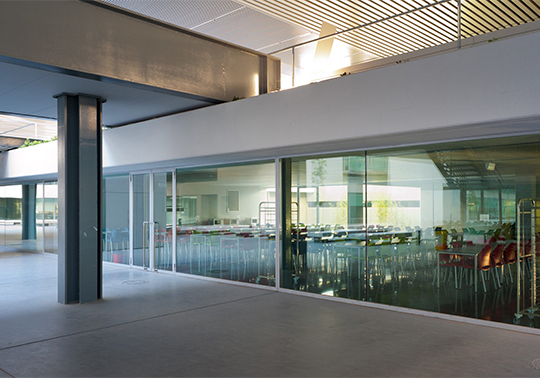
Miguel Rodrigo Bort, Professor at the ETSE-UV and researcher of cardiac arrhythmias, has developed a work together with A.J. Rogers, Prash Ganesan, M.I. Al-Husseini, C. Krittanawong and S.M Narayan together with the University of Stanford where he has implemented Machine Learning to separate atrial fibrillation from other arrhythmias.
The project was submitted to the American College of Cardiology’s Young Investigator Award, where it received an honourable mention. The work is motivated by the fact that cardiac arrhythmias, in particular atrial fibrillation (AF), are a major medical challenge. It compares different classifiers to separate AF from other cardiac arrhythmias. The study implies a profound advance in atrial fibrillation, as it provides advanced techniques for its automatic identification that can be extended to other clinical uses such as pacemakers or defibrillators.
To take forward the project, they compared classical detection methods based on clinical features with those based on Machine Learning (specifically, neural networks). These computational methods can classify the electrocardiographic signal without first extracting clinical features. "We found that both scoring systems, when trained with hundreds of thousands of signals (...), provide very good results," says Miguel Rodrigo, who adds that these results are similar in classification (AUC = 0.95).
Even so, one of the problems when introducing these types of classifiers based on deep learning in clinical practice is the refusal of experts, who are reluctant due to their lack of knowledge of the classification band. It should be noted that the learning materialises as numerical weights in internal classifier layers. This has been described on several occasions as 'black boxes' whose precise behaviour is unknown.
"With the aim of 'opening' this black box, we set out to create a series of experiments that would allow us to describe based on which specific features these deep learning classifiers were identifying AF patients," continues Miguel Rodrigo.
In pursuit of this goal, they created a database of 'reconstructed' signals, where clinical parameters of the signal were modified individually. At this point, they subjected this 'reconstructed' database to classification by the trained neural networks. The team weighted their output to see how these changes affected the classification provided by the neural network.
Following this scheme, the team was able to show that the classification provided by the neural networks was based on clinical parameters such as activation rate, the similarity between beats or their periodicity. "These controlled experiments have allowed us to define how deep learning-based classifiers identify AF, and also to provide concrete parameters," concludes Miguel Rodrigo.